How did we get here?
PredictionHealth was founded with the understanding that healthcare is broken because of a massive data problem. Huge amounts of data are collected for millions of individual patients every day, but harnessing and managing that data to deliver better patient care has been an ongoing problem for the healthcare industry. The COVID-19 pandemic has only made that issue more alarming.
We’ve been there. As providers we scribble and type notes, click checkboxes, pass messages along, and scrawl out little boxes on post-it notes to track our to-do lists. We get just enough into the Electronic Health Record (EHR) in a format barely suitable to justify billing, much less make a meaningful, actionable picture of how each encounter fits into the patient’s entire health journey.
COVID-19: A case study
It’s no surprise that when disaster struck in the form of a new virus COVID-19, we were left to stutter-step along, months behind the curve. Why? Because so much patient data goes ignored, uncaptured, undocumented, or unorganized leaving it difficult to understand and use.
The Journal of Thrombosis and Haemostasis published a paper on February 19, 2020 examining the link between novel coronavirus patients with a poor prognosis and abnormal coagulation parameters (1). And yet the realization that COVID-19 can cause death by blood clots was described as a “slow collective awakening.” (2) (Coagulation is set off by multiple potential etiologies and results in PEs, stroke, “COVID-toes”, and some clots so severe they result in the amputation of limbs).
With any reasonable tracking and real-time data assessment, we could better identify trends, disseminate information, and implement life-saving interventions by the time hundreds of patients are infected, not after tens of thousands of patients have already died. When death and loss of limb threaten millions of Americans, a “slow collective awakening” to facts that could save lives is unacceptable.
Another example is anosmia — an unusual but surprisingly prevalent symptom in up to 58% of COVID-19 cases that was mentioned in academic papers as early as March 28, 2020 and added by the CDC to the official lists of symptoms at the end of April, 2020 — a month later (3, 4, 5). In that time, the global case count had more than quadrupled, from 575,425 to 2,621,635, and the death toll increased from 26,628 to 182,097 (6). We are eventually likely to have another novel virus worse than COVID-19 and our healthcare system should be ready.
We should learn from other industries
This is not a new state of affairs. Healthcare providers have been held back by a patchwork system of misaligned incentives and madness that has been primarily increasing costs while leaving health and life expectancy to languish. We can do better.
And the first step to improvement is utilizing metrics and tracking. We have to be able to see where to course-correct in order to drive progress. COVID-19 has brought this into sharp focus.
Ideally the EHR systems could intelligently aggregate and share data so trends are rapidly identified and information disseminated to continually improve patient care and outcomes. It’s what internet companies have done with our data for years to improve their ad targeting and conversion — to get consumers to buy more stuff and spend countless hours responding to or liking content on social networks (7). How has so much time elapsed without the common use of machine learning to improve the health and wellbeing of our families and ourselves?
A new model, built around conversations
Imagine a small group of doctors has made a potential discovery — a new potential treatment or a useful symptom to check while triaging patients. How could you efficiently disseminate this to the 18 million healthcare workers (in the U.S. alone) and ensure they remember it and factor it in when a sick and scared patient is sitting in front of them(8)? A memo? Commercials? Email blasts to all workers across all 6,000 hospitals, to say nothing of the thousands of healthcare clinics, clinicians’ offices, and various health organizations (9)? The scope of the healthcare industry in this country is such that none of this is feasible with the current systems.
This is where PredictionHealth comes in. We’ve dedicated ourselves to capturing and structuring the data in real-time. We do this by processing all speech from patient encounters coming through our system to look for symptoms, diseases, and treatment mentions. This minimizes distraction during the clinician-patient interaction and frees the clinician to focus more on the patient while keeping the data secure and confidential. The other immediate benefit is that we can free up clinicians from the drudgery of documentation. Their notes are done for them – no extra after-hours dictation, no typing away furiously at the computer while the patient looks on. This saves our clinicians 2-3 hours per day, while freeing them to focus on each patient.
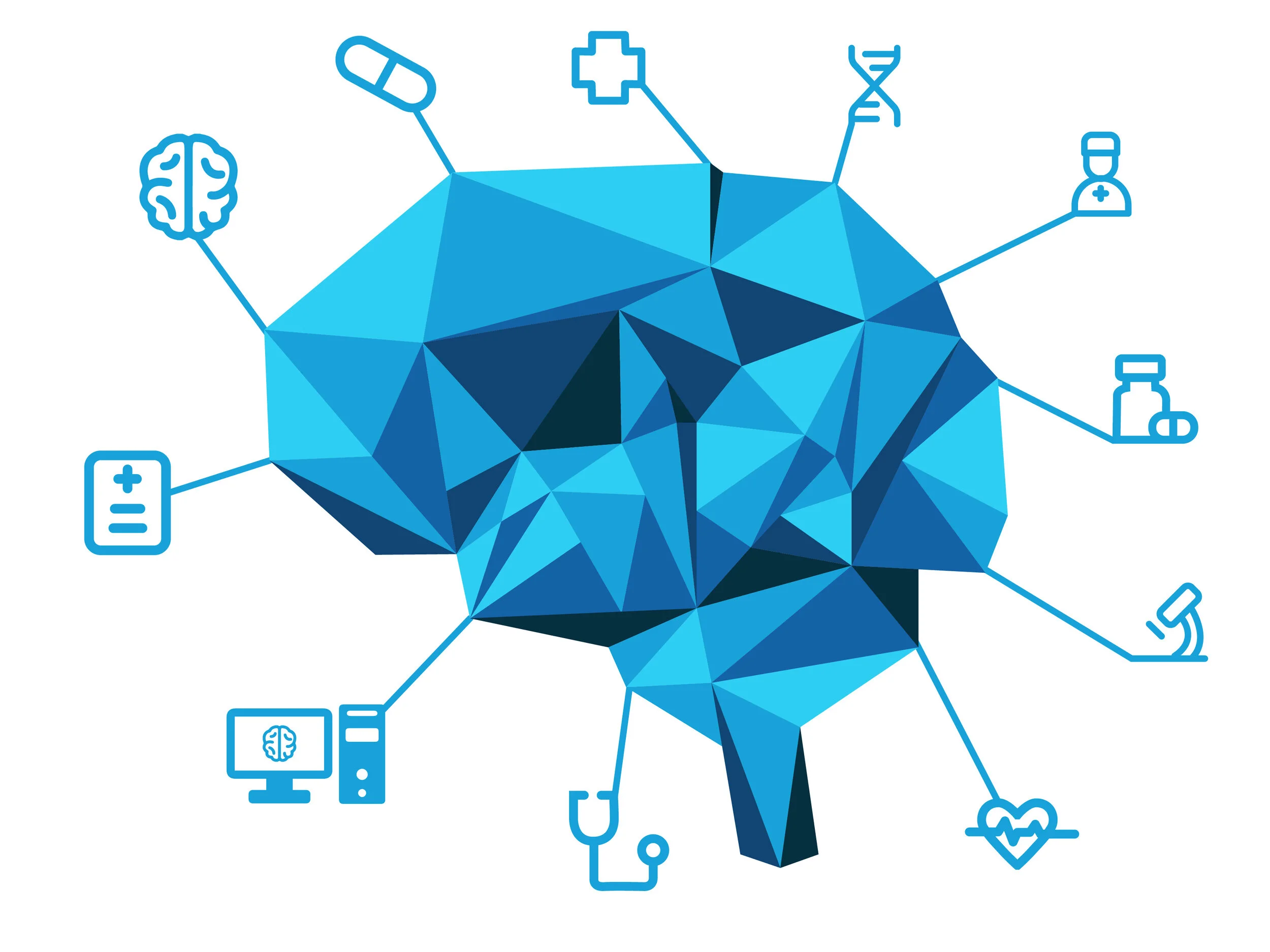
But we also take the real-time conversation data a step further by converting it into structured information streams we can leverage to develop custom models and understand what’s happening during each part of the clinical encounter. We currently apply this to improve our system’s efficiency but our prevailing goal is to provide the infrastructure that is desperately needed for the smarter healthcare we all deserve. We could detect and monitor seasonal disease trends like the flu. We could rapidly target and alert clinicians relevant information in real-time, or even integrate phenotyping algorithms and models that can be run for every single patient and every single conversation.
Supercharged research
Healthcare providers try to rely on robust studies and proven treatments when making most patient decisions, but even seemingly “strong” evidence can be misleading. Now, however, we are faced with a novel coronavirus, one for which we don’t have years of research or proven treatments. This forces clinicians to look to anecdotes shared by clinicians in unofficial channels such as Twitter, Facebook, and WhatsApp. A repository of preprint papers has been made available as well, but this requires research and assimilation of information across over 60,000 papers with 2,000 to 4,000 new ones added each week (as of Oct 2020) (10).
And the divide between available knowledge and actionable knowledge will persist beyond this pandemic. Half of medical “facts” go out of date every five years (11). New medical literature emerges at the rate of 2.5 million papers per year (12).
Want to scan across patient records to search for useful associations in the patchwork of EHR systems? You’re going to need a truckload of coffee. Each question can take months of work. We know because we’ve been working on this problem for years and published papers doing just that. We appreciate having healthcare data in a digital format, but there is no denying the difficulties in utilizing that data to improve care.
The good news
There is another “collective awakening” going on in the midst of the pandemic – to the exigency of transitioning current systems and inventing new ones that can manage and leverage data in smarter ways. We’re on that path, and working toward a solution that understands patient-clinician conversations to seamlessly incorporate AI into clinical workflows. Even though the problem of harnessing healthcare data may seem bigger than ever, at least we see it more clearly than ever, and we can all work together to bring the blaze under control.
What do you wish your EHR could predict? Let us know or get in touch to learn more about PredictionHealth.
-
Abnormal coagulation parameters are associated with poor prognosis in patients with novel coronavirus pneumonia | Journal of Thrombosis and Haemostasis
-
What the Coronavirus Crisis Reveals About American Medicine | The New Yorker
-
The COVID-19 Pandemic and Otolaryngology: What It Comes Down To? | PubMed
-
Loss of taste and smell is best indicator of COVID-19, study shows | The Harvard Gazette
-
C.D.C. Adds New Symptoms to Its List of Possible Covid-19 Signs | The New York Times
-
The National Institute for Occupational Safety and Health (NIOSH) | CDC
-
LitCovid | NIH
-
Thoughts for new medical students at a new medical school | BMJ
-
An overview of scientific and scholarly journal publishing | STM